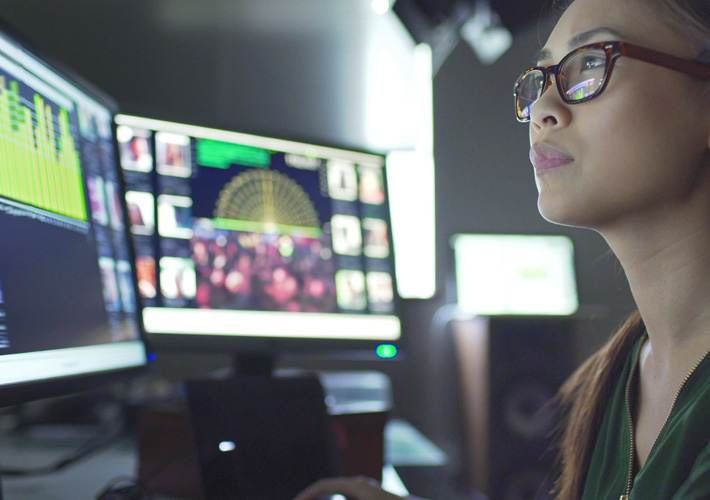
Revisiting Statistical Intervals: Common Statistical Intervals, Interval Selection, and Examples
Q: Can I infer something about a population or process based on a point estimate?
A: It is risky to use a point estimate based on a single sample of data to infer something about a population or process since it does not account for sources of inherent variation. Consider, for example, using the sample mean as a point estimate of the population mean. If multiple data samples of the same size were drawn under the same conditions from a population, the resulting sample means would all be different because the values making up each data sample would be different. This is an illustration of uncertainty due to sampling. There may be other sources of variability as well, such as those due to environmental effects or measurement error.
Statistical intervals are used to account for uncertainty in a point estimate based on a single sample of data, which is standard practice. Though statistical intervals can be applied across a wide variety of applications, it is a topic that is not generally well understood.1 This article combines information from previous installments of Data Points to provide a high-level overview of the common types of statistical intervals, interval selection, and example interval formulations.2
Common statistical intervals are the confidence interval (CI), prediction interval (PI), and tolerance interval (TI). Interval selection depends on the purpose of the interval and the statistic of interest.3 A CI is used to make an inference about an unknown parameter, such as a mean, a standard deviation, or a proportion. A PI is used when the interest is in predicting bounds for one or more future observations. A TI is used to capture a specified proportion of the population. Parametric methods (using an assumed distribution) and nonparametric methods (distribution-free) can be used to construct each interval type. Both parametric and nonparametric methods assume that the sample is a random representation of a population or stable process.4
Statistical intervals can be one-sided or two-sided. For example, a two-sided CI is typically used when making an inference about the mean, whereas a one-sided lower CI is often used when estimating reliability. All intervals have a confidence level, which represents the belief (as a percent probability) that the method used to construct the interval has correctly captured the truth. The confidence level is specified in advance for parametric methods, commonly 95%. For nonparametric methods it is a function of the sample size (and proportion for a TI).
The data in Table 1 is used in Table 2 to provide examples of two-sided CI, PI, and TI that assume a normal distribution and non-parametric PI and TI counterparts.5
For parametric intervals, in general and as observed in Table 2, a PI will always be wider than a CI given the same level of confidence and sample size. Note that with a sample size of n = 22, the confidence levels for the nonparametric PI and TI are 91% and 66%, respectively.
Though there is no singe correct method for constructing a given type of interval, it is important to understand advantages and/or limitations of available methods. If normality does not hold, then a nonparametric method may be a better option. However, it would take at least n = 39 and n = 46 for the nonparametric PI and TI, respectively, to achieve the equivalent 95% confidence level versus the example sample size of 22.
For the most comprehensive information on statistical intervals, the reader is encouraged to see Statistical Intervals: A Guide for Practitioners and Researchers.6
REFERENCES
1 Luko, S.N. (Editor). Presentation of Data and Control Chart Analysis (“Manual 7”). Ninth Edition. ASTM International, 2018.
2 Luko, S.N. and Neubauer, D.V. “Statistical Intervals Part 1: The Confidence Interval.” Standardization News (July/Aug. 2011): 18-20; “Statistical Intervals Part 2: The Prediction Interval.” Standardization News (Sept./Oct. 2011): 14-15; “Statistical Intervals Part 3: More on the Tolerance Interval.” Standardization News (Nov./Dec. 2011): 18-19; “Statistical Intervals: Nonparametric Intervals Part 1.” Standardization News (Nov./Dec. 2013): 20-21; “Statistical Intervals: Nonparametric Intervals Part 2.” Standardization News (Jan./Feb. 2014): 20-21.
3 Luko, S.N. Presentation of Data and Control Chart Analysis.
4 Ibid.
5 Luko, S.N. and Neubauer, D.V. “Statistical Intervals Part 1: The Confidence Interval.”
6 Meeker, W.Q., Hahn, G.J., and Escobar, L.A., Statistical Intervals: A Guide for Practitioners and Researchers. Second Edition. John Wiley & Sons, Inc., 2017: (103-104).
7 Ibid.; Wilks, S.S. Mathematical Statistics. New York: John Wiley & Sons, 1962.
Stephen N. Luko is a retired statistician with 40 years of industrial experience, holding the title of Fellow at Collins Aerospace, ASTM International, and the American Society for Quality. He is currently chair of the subcommittee on reliability (E11.40) and is past chair of the committee on quality and statistics (E11).
Jennifer Brown is a statistician with 16 years of experience in the aerospace industry. She is chair of the subcommittee on terminology (E11.70) and a member of the subcommittee on specialized NDT methods (E07.10).
John Carson, Ph.D., is senior statistician for Neptune and Co and the Data Points column coordinator. Carson is a member of the committees on quality and statistics (E11), petroleum products, liquid fuels, and lubricants (D02), air quality (D22), and environmental assessment, risk management, and corrective action (E50).